Harnessing Artificial Intelligence: Analyzing FASB Comment Letters on the Disaggregation of Income Statement Expenses
This article delves into the use of Natural Language Processing (NLP) to analyze Financial Accounting Standards Board (FASB) comment letters, focusing on the Disaggregation of Income Statement Expenses (DISE).
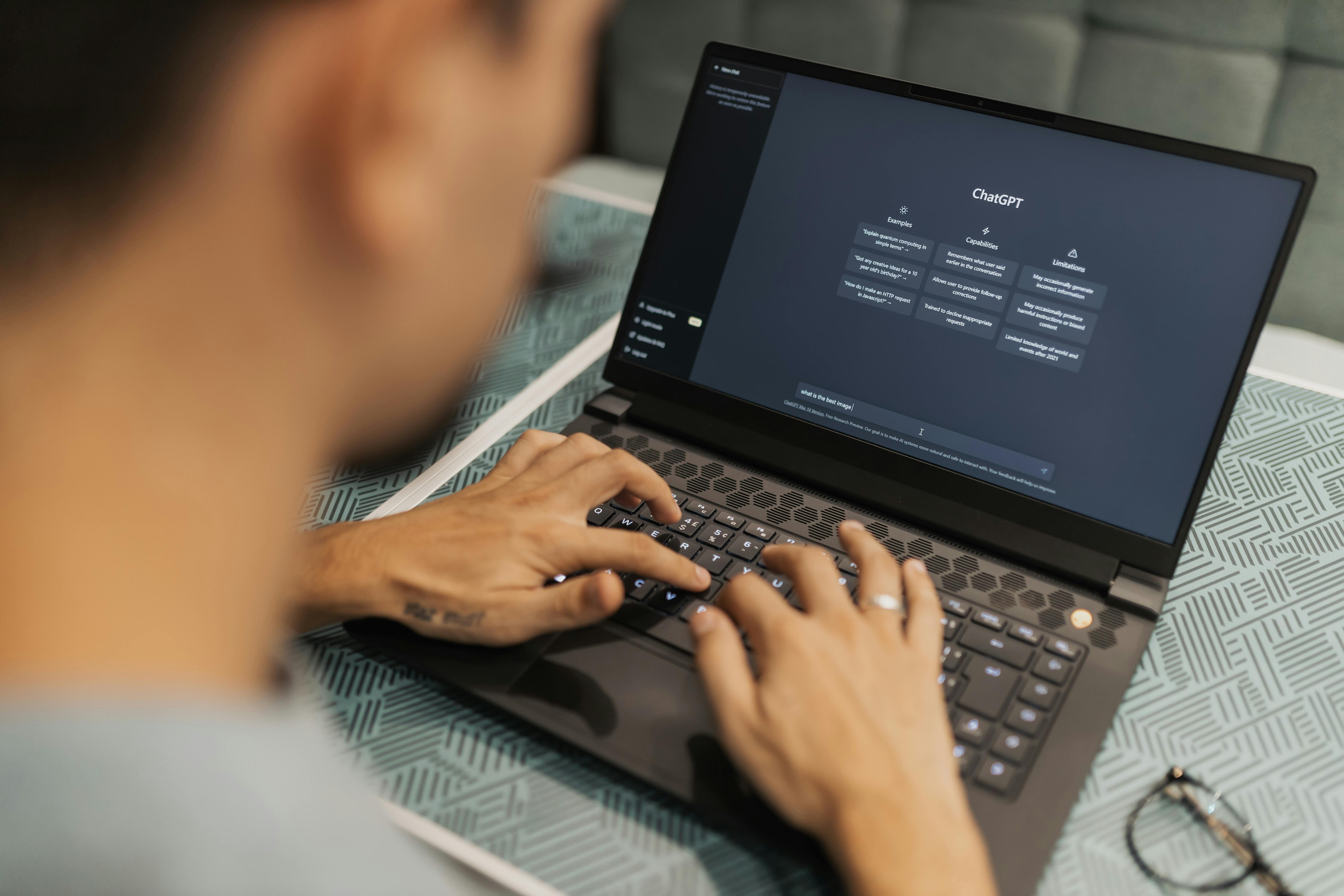
Abstract
The complexity of accounting standards and the imperative for stakeholder engagement have
elevated the importance of comment letters in shaping financial regulations. However, traditional
analysis of these letters is time-consuming and prone to human bias. This study explores the
application of Artificial Intelligence (AI), specifically Natural Language Processing (NLP), to
analyze comment letters related to the Financial Accounting Standards Board's (FASB) project
on the Disaggregation of Income Statement Expenses (DISE). By leveraging AI tools, we aim to
enhance the efficiency, objectivity, and depth of analysis, thereby aligning accounting research
with advanced technological methodologies.
Introduction
The evolution of accounting standards necessitates rigorous stakeholder engagement to ensure
that new regulations meet the diverse needs of preparers, investors, and other interested
parties. Comment letters submitted to the Financial Accounting Standards Board (FASB) offer
invaluable insights into stakeholder perspectives on proposed changes. Traditionally, analyzing
these letters involves manual review, which is both labor-intensive and susceptible to bias.
Recent advancements in Artificial Intelligence (AI), particularly in Natural Language Processing
(NLP), present opportunities to revolutionize this analytical process. AI can process vast
amounts of textual data rapidly and objectively, uncovering patterns and insights that might be
overlooked in manual analyses. This research focuses on utilizing AI to analyze comment letters
pertaining to the FASB's "Disaggregation of Income Statement Expenses" (DISE) project.
Purpose and Scope
The primary objective of this study is to demonstrate how AI can effectively extract, compare,
and synthesize insights from stakeholders' responses to the DISE project. By doing so, we aim
to:
- Enhance Analytical Efficiency: Streamline the analysis of extensive textual data.
- Improve Objectivity: Minimize human bias in interpreting stakeholder feedback.
- Contribute to Accounting Research: Showcase AI's potential in advancing research methodologies within the accounting discipline.
Methodology
Data Collection
We collected all publicly available comment letters submitted to the FASB regarding the DISE
project. These letters represent a diverse array of stakeholders, including:
• Corporate Preparers
• Investors
• Academics
• Industry Associations
• Regulatory Bodies
Analytical Framework
1. Batch Processing
To manage the substantial volume of data, we employed batch processing, analyzing the letters
in groups of ten. This approach allowed for systematic and organized analysis.
2. Structured Analysis
Each batch underwent a comprehensive analysis consisting of:
- Sensitivity Analysis: Assessing how varying assumptions and variables influence each stakeholder's stance.
- Key Highlights Extraction:
- Objectives and Findings: Summarizing the main points of each letter.
- Core Assumptions or Variables: Identifying underlying premises affecting opinions.
- Strengths, Weaknesses, and Uncertainties: Evaluating the robustness of arguments presented.
- Tone Assessment: Assigning descriptors (e.g., supportive, critical, neutral) to gauge sentiment.
- Comparative Analysis: Identifying similarities and differences across letters, focusing on methodology, tone, and key findings.
- Trend Synthesis: Highlighting recurring themes, stakeholder conflicts, and industry-specific challenges.
3. Data Documentation
Findings were meticulously documented in both spreadsheet and narrative formats to facilitate
cross-referencing and in-depth analysis.
AI Tools
We utilized ChatGPT 4o for its advanced NLP capabilities, which include:
- Text Summarization: Efficient extraction of key points.
- Sentiment Analysis: Detection of emotional tone and bias.
- Pattern Recognition: Identification of trends and commonalities across documents.
Findings and Analysis
Key Insights and Overarching Themes - DISE
1. Transparency Versus Implementation Burden
• Support for Transparency: An overwhelming majority acknowledged the value of
increased transparency in financial reporting to aid investor decision-making.
• Implementation Concerns: Many preparers highlighted significant operational
challenges, including system overhauls, increased administrative costs, and the need for
staff training.
2. Cost-Benefit Considerations
• Investors and Academics: Emphasized the long-term benefits of detailed disclosures,
such as improved market efficiency and investor protection.
• Corporate Preparers: Focused on immediate costs and resource allocation, questioning
whether the benefits justify the expenses involved.
3. Industry-Specific Challenges
• Manufacturing and Aerospace: Pointed out complexities in cost allocations due to
intricate supply chains and production processes.
• Retail and Real Estate: Argued that disaggregated expense information may not
significantly enhance the understanding of their financial statements.
• Insurance and Extractive Industries: Noted that their unique business models and
regulatory environments might render the proposed standards less applicable or
burdensome.
4. Calls for Tailored Approaches
• Materiality Thresholds: Suggestions to include thresholds to prevent immaterial
disclosures that could clutter financial statements.
• Phased Implementation: Proposals for gradual adoption to allow entities time to adjust
systems and processes.
• Industry-Specific Guidelines: Recommendations for the FASB to consider sector-specific
reporting requirements.
Similarities and Differences Across Stakeholders
Common Ground
• Universal Support for Enhanced Transparency: All stakeholders agreed on the
importance of transparency for investor decision-making.
• Recognition of Operational Challenges: Acknowledgment of the practical difficulties in
implementing new reporting standards.
Divergent Views
• Tone: Investors and academics generally used a supportive tone, while corporate
preparers were more critical.
• Scope of Application: Debate over whether the standards should apply to both public
and private entities.
• Degree of Disaggregation: Varied opinions on the level of detail required, with some
advocating for high-level summaries and others for granular disclosures.
Trends and Patterns
• Demand for Standardization: A call for clear definitions to ensure consistency and
comparability across financial statements.
• Emphasis on Materiality: A trend toward advocating for disclosures that focuses on
material information to avoid information overload.
• Risk of Competitive Harm: Concerns that detailed disclosures could reveal proprietary
information, potentially disadvantaging companies competitively.
Discussion
Implications of AI in Accounting Research
1. AI as a Catalyst for Efficiency
• Rapid Processing: AI significantly reduces the time required to analyze large volumes of
data.
• Resource Optimization: Allows researchers to allocate time to higher-level analysis
rather than data gathering and preliminary assessments.
2. Enhancing Objectivity
• Bias Mitigation: AI provides consistent evaluation criteria, reducing the influence of
individual biases.• Comprehensive Coverage: Ensures all documents are analyzed uniformly, capturing
insights that might be missed in selective manual reviews.
3. Scalability and Replicability
• Applicability Across Projects: The methodology can be applied to other accounting
standards and regulatory analyses.
• Foundation for Future Research: Establishes a framework that can be refined and
expanded upon in subsequent studies.
Challenges and Limitations
• Contextual Understanding: AI may misinterpret nuanced language or industry-specific
terminology without proper training.
• Quality of Input Data: Inconsistencies in comment letter formats and content can affect
the accuracy of AI analysis.
• Ethical Considerations: Reliance on AI necessitates transparency in methodology to
maintain credibility and trust in the findings.
Conclusion
This study underscores the transformative potential of AI in accounting research, particularly in
analyzing stakeholder feedback on regulatory proposals. By employing AI tools to process
comment letters on the FASB's DISE project, we demonstrated enhanced efficiency, objectivity,
and depth in our analysis. The findings reveal widespread support for increased transparency,
tempered by practical concerns over implementation burdens and industry-specific challenges.
As the accounting profession continues to evolve, integrating AI into research methodologies
offers a pathway to more robust and insightful analyses. Future research should focus on
improving AI's contextual understanding and addressing ethical considerations. Embracing AI
not only modernizes accounting research but also contributes to more informed and balanced
standard-setting processes.
Appendix
ChatGPT 4o Prompts
1. Article Analysis Prompt
Task: Analyze and compare 10 documents by performing a sensitivity analysis on each.
Provide highlighted information summarizing key points to facilitate effective comparison
between the documents.
Instructions For Each Document:
Sensitivity Analysis: Identify how changes in key variables or assumptions affect the outcomes
or conclusions of the document.
Key Highlights:
• Summarize the main objectives and findings.
• Note any critical data, assumptions, or variables used.
• Highlight strengths, weaknesses, and any areas of uncertainty.
• Give a one-word answer for the tone of the document in a range (Highly Positive,
Positive, Neutral, Negative, Highly Negative)
Comparison Across Documents:
• Identify similarities and differences in methodologies, assumptions, and conclusions.
• Highlight any conflicting data or perspectives.
• Provide insights into trends or patterns observed across the documents.
Presentation:
• Organize the information in a clear and structured table that has the column headings
(Document, Main Objective & Findings, Key Variables/Assumptions, Strengths,
Weaknesses, Uncertainty, Tone)
• Use headings and subheadings for easy navigation.
• Emphasize significant findings using bold or italics.
2. Findings Analysis Prompt
Please analyze the attached spreadsheet and Word document containing AI findings on FASB
Comment Letter Articles. The Word document highlights similarities, differences, trends, and
patterns identified by the AI for each set of articles. The spreadsheet lists findings from each
article categorized under Document, Main Objective & Findings, Key Variables/Assumptions,
Strengths, Weaknesses, Uncertainty, and Tone.
I would like you to provide a detailed analysis of the information from both documents. Please:
• Summarize the key insights and overarching themes identified.
• Discuss significant observations regarding similarities and differences among the
articles.
• Highlight any notable trends and patterns found by the AI.
• Synthesize the data to present comprehensive findings suitable for inclusion in my
accounting research article about the use of AI in analyzing FASB Comment Letter
Articles.